CHALLENGES
The client had multiple data that was cluttered, incomplete and was creating hinderance in the training of Machine Learning. The client needed a solution in the form of synthetic data which could be useful for them.
SOLUTIONS
After carefully considering the client’s requirements and conducting a comprehensive evaluation of available tools, Euphoric’s team decided to use a Machine Learning algorithm-based code, named ‘Faker’ to solve this problem. Faker is a Python package that generates fake data.
BENEFITS
With this approach, the client was able to achieve the following benefits:
- Privacy Protection: Synthetic data got used as a substitute for real patient data, which reduced the risk of privacy breaches and ensuring compliance with data protection regulations.
- Rare Cases & Simulating Clinical Scenarios: Synthetic data generation helped to create synthetic instances of rare diseases, unusual conditions, or outlier cases. This allowed the client to train models that are better equipped to detect and handle such cases. It also helped to simulate various clinical scenarios, treatments, and interventions. This enabled the client to test and refine treatment protocols, evaluate the effectiveness of different strategies, and conduct virtual experiments without involving real patients.
- Algorithm Development and Testing: By creating synthetic datasets with known ground truth labels, the client got to assess the performance and limitations of their algorithms in controlled settings before deploying them in real-world scenarios.
- Educational and Training Purposes: Synthetic data helped in simulating various patient cases, medical images, or clinical scenarios, allowing learners to gain practical experience without compromising patient privacy
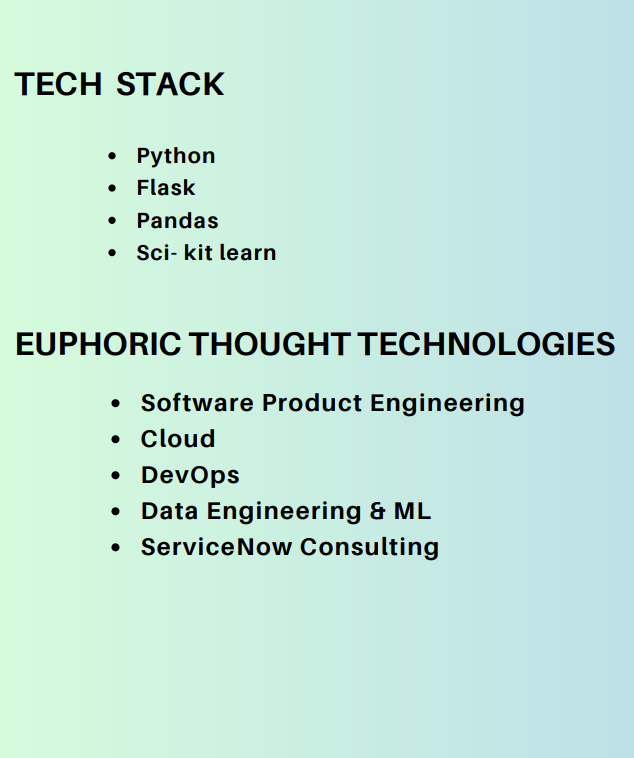